Stable Diffusion vs DALL·E 3: How They Differ from Each Other
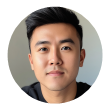
Updated on
As both Stable Diffusion and DALL·E 3 are pushing the boundaries of what's possible in generative AI, a natural question arises: which one reigns supreme? In this comprehensive comparison guide, we'll delve into the strengths, weaknesses, and unique capabilities of Stable Diffusion and DALL·E 3, exploring their model architectures, training methodologies, image generation capabilities, user experiences, ethical considerations, and performance characteristics.
Whether you're an artist seeking a powerful creative tool, a developer exploring the frontiers of AI, or simply a curious observer of this rapidly evolving field, this blog post will provide valuable insights into the capabilities and trade-offs of these two remarkable AI models.
Now let's start.
Stable Diffusion vs DALL·E 3: Model Architecture and Training
Stable Diffusion employs a latent diffusion model architecture, which is a type of generative model that operates in a compressed latent space. This approach involves learning to reverse the process of gradually adding noise to images during training. At the core of Stable Diffusion lies a U-Net convolutional neural network, which serves as the backbone for the diffusion model.
The training process for Stable Diffusion involves a large dataset of images from the internet, including the LAION-5B dataset with over 5 billion image-text pairs. The model is trained using denoising diffusion probabilistic models, which learn to generate images by reversing the noise addition process. By operating in a lower-dimensional latent space, Stable Diffusion achieves computational efficiency, making it capable of running on consumer-grade GPUs.
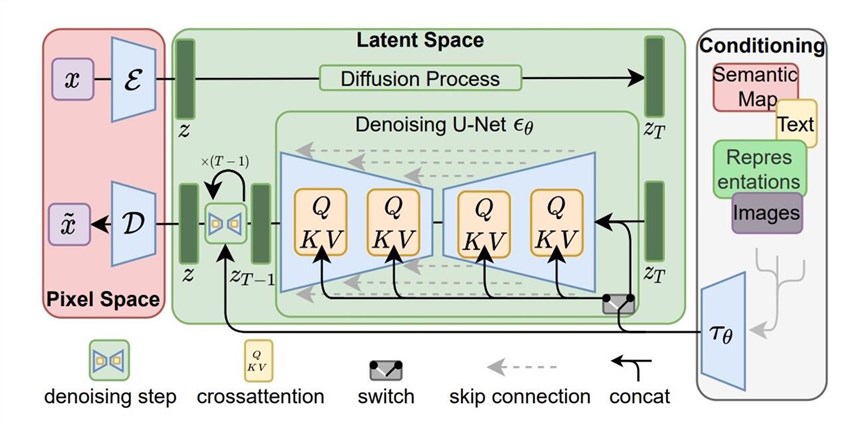
DALL·E 3, on the other hand, employs a transformer-based architecture similar to large language models like GPT-3. This architecture consists of an encoder-decoder structure, where the encoder processes the text prompt, and the decoder generates the corresponding image. The model leverages attention mechanisms to capture long-range dependencies and understand complex prompts effectively.
The training data for DALL·E 3 comprises a curated subset of images and their corresponding captions from the internet. OpenAI has focused on high-quality, carefully filtered data to ensure the model learns accurate relationships between text and visual representations. While the exact size of the training dataset and model parameters remain undisclosed, it is widely believed that DALL·E 3 is significantly larger and more resource-intensive than Stable Diffusion.
DALL·E 3's transformer-based architecture allows it to excel at understanding and interpreting nuanced text prompts, but this comes at the cost of higher computational requirements compared to Stable Diffusion's more efficient latent diffusion model approach.
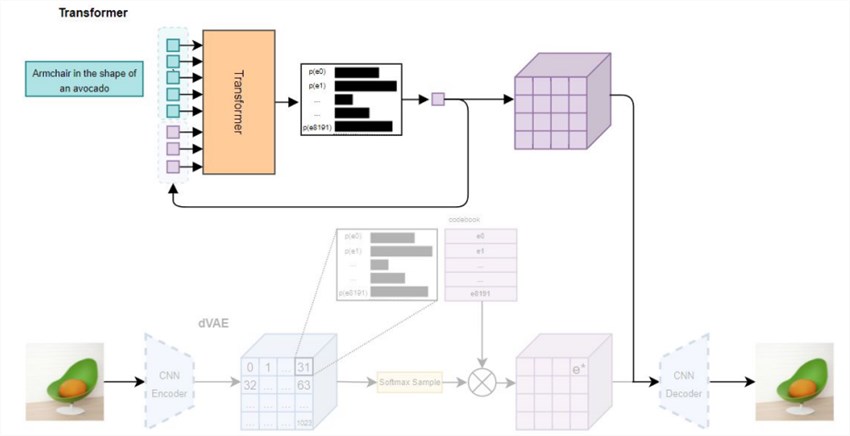
Stable Diffusion vs DALL·E 3: Image Generation Capabilities
Stable Diffusion has garnered significant praise for its ability to generate highly photorealistic and visually stunning images. The model excels at rendering complex scenes, intricate textures, and fine details with an impressive degree of realism. This is achieved through the model's training on a vast dataset of internet images, allowing it to capture and reproduce the nuances of real-world visuals effectively.
One of Stable Diffusion's strengths lies in its ability to handle multiple subjects and elements within a single image prompt. Users can describe elaborate scenes, and the model will attempt to coherently depict all the specified components, making it a powerful tool for creative visualization and artistic exploration. Additionally, Stable Diffusion offers a wide range of artistic styles, from photorealistic to stylized and abstract, catering to diverse creative preferences.
However, while Stable Diffusion's outputs are often visually striking, the model can sometimes struggle with accurately depicting specific concepts or following prompts to the letter, especially when dealing with complex scenes or instructions. This is where DALL·E 3 demonstrates its prowess in prompt comprehension and adherence.
DALL·E 3 has garnered significant attention for its exceptional ability to understand and accurately depict the nuances and specifics described in text prompts. Even when presented with complex scenes or intricate instructions, DALL·E 3 demonstrates a remarkable capacity to capture and render the intended visual elements with precision.
One area where DALL·E 3 particularly excels is in rendering text, logos, and other graphical elements within images. This capability makes it a valuable tool for tasks such as generating product mockups, designing logos, or creating visual representations of textual descriptions.
While DALL·E 3's outputs are highly coherent and aligned with the provided prompts, they can sometimes appear more stylized or computer-generated than the photorealistic outputs of Stable Diffusion. Additionally, DALL·E 3 may have a more limited range of artistic styles compared to the diverse outputs achievable with Stable Diffusion.
It's worth noting that Stable Diffusion allows users to generate multiple images from a single prompt, enabling them to choose the best output, while DALL·E 3 generates a fixed number of images per prompt (typically 4). Furthermore, Stable Diffusion offers more control over the image generation process, allowing users to adjust parameters like the number of steps, seed values, prompt strengths, and the ability to use negative prompts to exclude certain elements.
However, there is a big challenge for both Stable Diffusion and DALL·E 3, which is achieving high-quality, high-resolution images directly from the generation process. Often, the initial outputs can suffer from noise, lack of sharpness, and lower resolution, which can detract from the overall impact of your artwork.
Aiarty Image Enhancer addresses these pain points by leveraging advanced AI technology to upscale, denoise, and sharpen your images. This software brings out the finest details, enhances textures, and ensures your artwork looks polished and professional. Whether you're looking to refine intricate designs or simply add a professional touch to your images, Aiarty Image Enhancer provides the tools you need for stunning results. Transform your creations effortlessly and see the difference that professional-grade image enhancement can make.
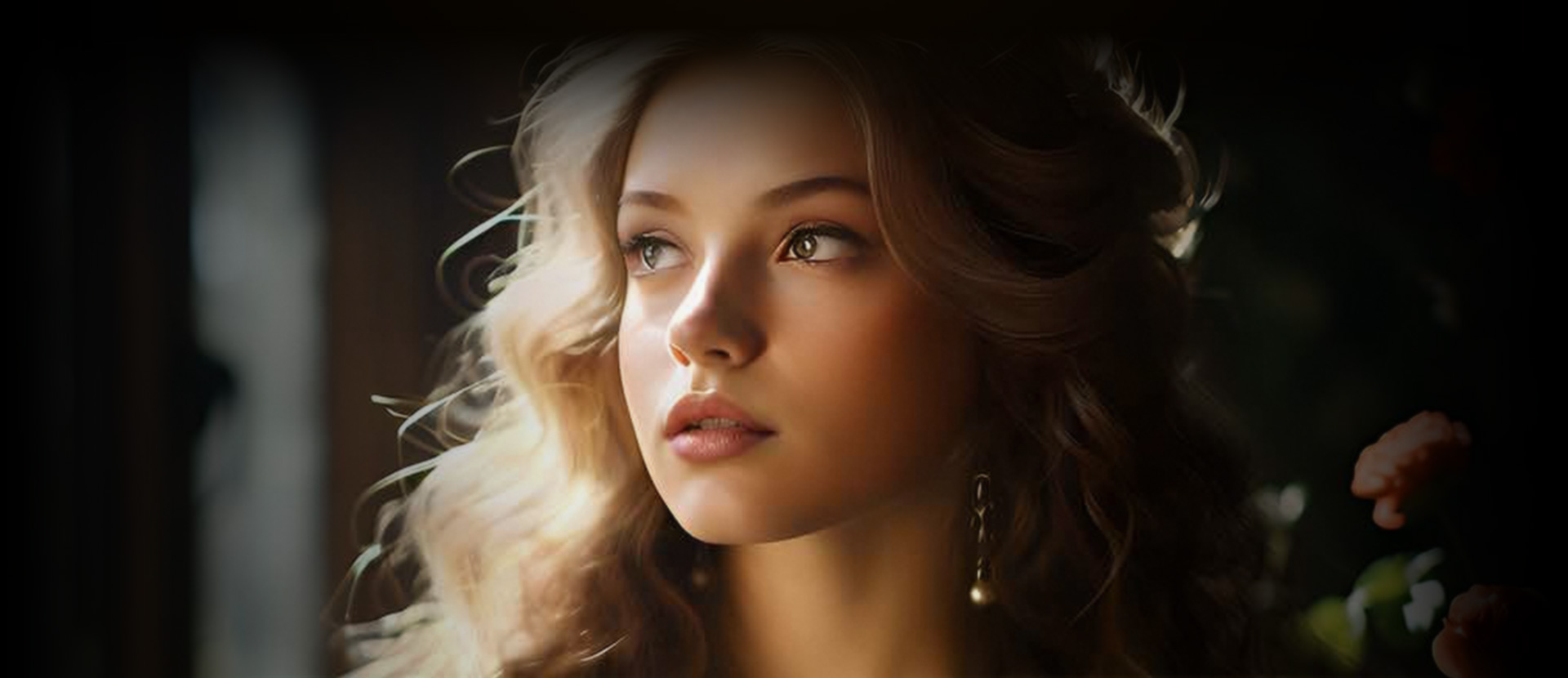
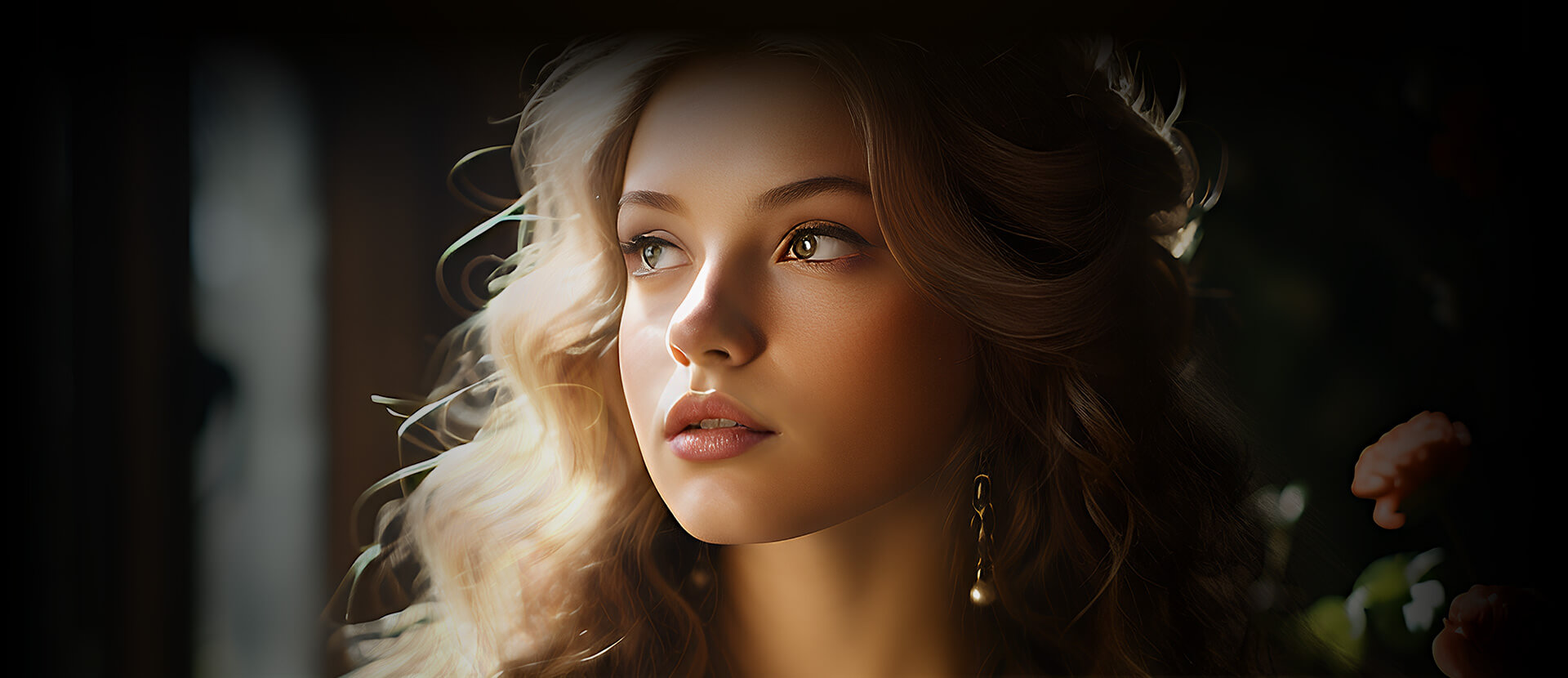
Stable Diffusion vs DALL·E 3: User Experience and Interactivity
Stable Diffusion, being an open-source model, offers users a high degree of flexibility and control over the image generation process. One of the most popular interfaces for Stable Diffusion is AUTOMATIC1111's WebUI, a browser-based application that allows users to input text prompts, adjust various generation parameters, and view the resulting images.
Another notable implementation is DreamStudio by Stability AI, which provides a more polished and user-friendly experience while still offering advanced features like image upscaling and inpainting. With Stable Diffusion, users have the ability to fine-tune a wide range of parameters, such as the number of inference steps, seed values, and prompt strengths, enabling them to achieve highly customized and tailored results.

A unique aspect of Stable Diffusion is its support for negative prompts, which allow users to exclude specific elements or styles from the generated images. This feature empowers users to exercise greater control over the output, ensuring that unwanted components are omitted from the final result.
Furthermore, Stable Diffusion offers advanced capabilities like inpainting and outpainting. Inpainting enables users to modify specific regions of an existing image, while outpainting allows them to extend the canvas beyond the original image boundaries. These features foster an iterative and interactive creative process, where users can continuously refine and build upon their generated images.
However, it's important to note that the open-source nature of Stable Diffusion can also present a steeper learning curve for some users. Navigating command-line interfaces or configuring various settings may require a certain level of technical proficiency, which could be a barrier for those seeking a more streamlined experience.
DALL·E 3 takes a different approach to user experience by offering a seamless integration with ChatGPT, the company's conversational AI assistant. Users can engage in a natural language dialogue, providing text prompts and receiving generated images in response.
Note: You can access DALL·E 3 through ChatGPT only when you are its Plus member. If you want to try it for free, you can use Bing's Copilot.

One of the standout features of DALL·E 3 is its exceptional prompt comprehension and adherence. Users can provide detailed and nuanced prompts, and the model will strive to accurately depict the described elements, making it highly intuitive for users who may not be familiar with advanced generation parameters.
The conversational interface of DALL·E 3 allows for an iterative refinement process, where users can provide feedback and additional prompts to refine the generated images. However, the level of control and customization is more limited compared to Stable Diffusion, as users cannot directly adjust generation parameters or utilize advanced features like inpainting or outpainting.
It's worth noting that DALL·E 3 is a closed, proprietary model offered as a service by OpenAI, which means users do not have direct access to the underlying model or the ability to modify it. This approach prioritizes ease of use and accessibility, making DALL·E 3 a more user-friendly option for those who prefer a streamlined experience without the need for extensive technical knowledge.
Stable Diffusion vs DALL·E 3: Performance and Scalability
One of the key advantages of Stable Diffusion is its scalability and customization potential. Being an open-source model, users and developers have the ability to extensively customize and fine-tune Stable Diffusion on specific datasets or tasks. This flexibility allows for the creation of specialized models tailored to specific domains or use cases, making Stable Diffusion an attractive choice for enterprises and developers seeking to leverage the power of generative AI.
Furthermore, the open-source nature of Stable Diffusion fosters a vibrant community ecosystem, where users can share and leverage pre-trained models from platforms like Civitai. This collaborative approach accelerates the development and refinement of Stable Diffusion models, further enhancing its scalability and adaptability.
Howerver, when it comes to computational requirements, Stable Diffusion models have relatively high demands, especially for generating high-resolution images. This is due to the iterative denoising process employed by the latent diffusion approach, which requires significant computational power. However, by operating in a compressed latent space, Stable Diffusion models are able to reduce computational demands compared to direct diffusion models that operate on the full image space.
Despite this optimization, Stable Diffusion still requires powerful hardware, such as high-end GPUs or TPUs, for efficient training and inference. The inference speed of Stable Diffusion can be a bottleneck, with typical inference times ranging from a few seconds to over a minute for high-quality outputs. Factors like the number of inference steps, batch size, and the capabilities of the underlying hardware can significantly impact the inference speed.
As a proprietary model developed by OpenAI, the exact computational requirements for DALL·E 3 are not publicly disclosed. However, given its transformer-based architecture and large model size, it is expected to have significant computational demands, likely requiring specialized hardware such as TPUs for efficient inference.
But one area where DALL·E 3 excels is inference speed. The model has demonstrated impressive performance, generating high-quality images in a matter of seconds. This speed advantage over iterative diffusion models like Stable Diffusion can be attributed to DALL·E 3's transformer-based architecture and optimized inference pipeline.
However, the trade-off for this speed comes in the form of limited customization and scalability options. As a closed, proprietary model offered as a service by OpenAI, users do not have the ability to directly customize or fine-tune DALL·E 3, which we have talked about before a few times. While OpenAI may periodically release updated versions of the model with improved capabilities, users have limited control over the underlying architecture or training process.
The scalability of DALL·E 3 is primarily determined by OpenAI's infrastructure and resource allocation for the service. Users are essentially reliant on OpenAI's roadmap and investment in scaling the model's capabilities, rather than having the flexibility to scale and customize the model themselves.
Over to You
We have pretty much covered all the differences between Stable Diffusion and DALL·E 3, showing you their both strengths and weaknesses. Now it's your turn to make your choice between them. So which one would you go for?